In today’s rapidly evolving technological landscape, the convergence of Edge AI and cloud computing is reshaping how we process and analyze data. Edge AI empowers devices to perform real-time data processing right where the data is generated, significantly reducing latency and enhancing operational efficiency. By handling computations locally, it alleviates the burden on cloud systems, allowing them to focus on aggregating and analyzing vast amounts of data over time. This synergy not only improves network efficiency but also paves the way for more robust, scalable solutions, driving innovation across industries.
In today’s digital landscape, the synergy between Edge AI and cloud computing presents remarkable opportunities for enhancing efficiency and responsiveness. By processing data nearer to its source, Edge AI minimizes latency and optimizes the performance of cloud infrastructure. This article delves into the mechanisms that underscore this dynamic relationship and outlines the benefits it offers across various sectors.
The Convergence of Edge AI and Cloud Computing
The intersection of Edge AI and cloud computing is crucial for modern applications that demand real-time processing. Edge AI empowers devices to handle data at the source, while the cloud takes on the role of managing, analyzing, and storing vast datasets. This partnership ensures that only essential information is transmitted to the cloud, effectively streamlining operations and improving overall network efficiency. The combination allows organizations to harness real-time capabilities together with comprehensive analytics, thereby amplifying both immediate response and long-term insights.
How Edge AI Improves Data Processing
One of the primary advantages of Edge AI is its ability to process data locally, which significantly reduces the amount of information that needs to be sent to the cloud. By utilizing edge computing principles, devices become adept at handling tasks that once required remote cloud resources. This local processing capability not only enhances the efficiency of data handling but also leads to considerable energy savings on operational costs. Devices embedded with Edge AI are designed for optimum power consumption, making them an eco-friendly choice for organizations seeking sustainable solutions. For more on this, you can learn about the benefits laid out in this beginner’s guide.
Real-Time Decision-Making Capabilities
With Edge AI, organizations can achieve ultra-low latency, enabling instantaneous decisions based on the most recent data. Edge devices are able to analyze sensor data and respond to changes in real time without waiting for cloud processing. This is particularly beneficial in areas such as industrial automation and healthcare monitoring, where timely actions are critical. Meanwhile, the cloud plays a vital role in accumulating and analyzing historical data, leading to deeper insights over time. Together, they create a robust framework that enhances operational responsiveness. Explore more about this synergy in detail through the insights provided in this article.
Optimizing Bandwidth and Cost Efficiency
By processing data closer to its source, Edge AI contributes significantly to bandwidth optimization. Reducing the amount of data sent to the cloud lowers costs associated with data transmission and storage. Application examples include autonomous vehicles and smart city solutions, where Edge AI minimizes the need for continuous cloud connectivity. Moreover, this efficiency leads to less congestion over networks and ensures that only actionable data is passed to cloud services, enabling a leaner operation. To explore the impact further, refer to this insightful resource.
Future-Ready and Hybrid Solutions
As businesses continue to scale, the need for future-ready and hybrid solutions becomes critical. Edge AI and cloud computing enable companies to design their architectures flexibly, allowing them to seamlessly adapt to changing demands. The rising adoption of smart devices and IoT applications necessitates a framework that can handle diverse data loads with agility. By incorporating Edge AI, organizations can future-proof their operations while also maintaining cost-effectiveness. For an in-depth understanding of how edge computing complements cloud strategies, check out this explanation.
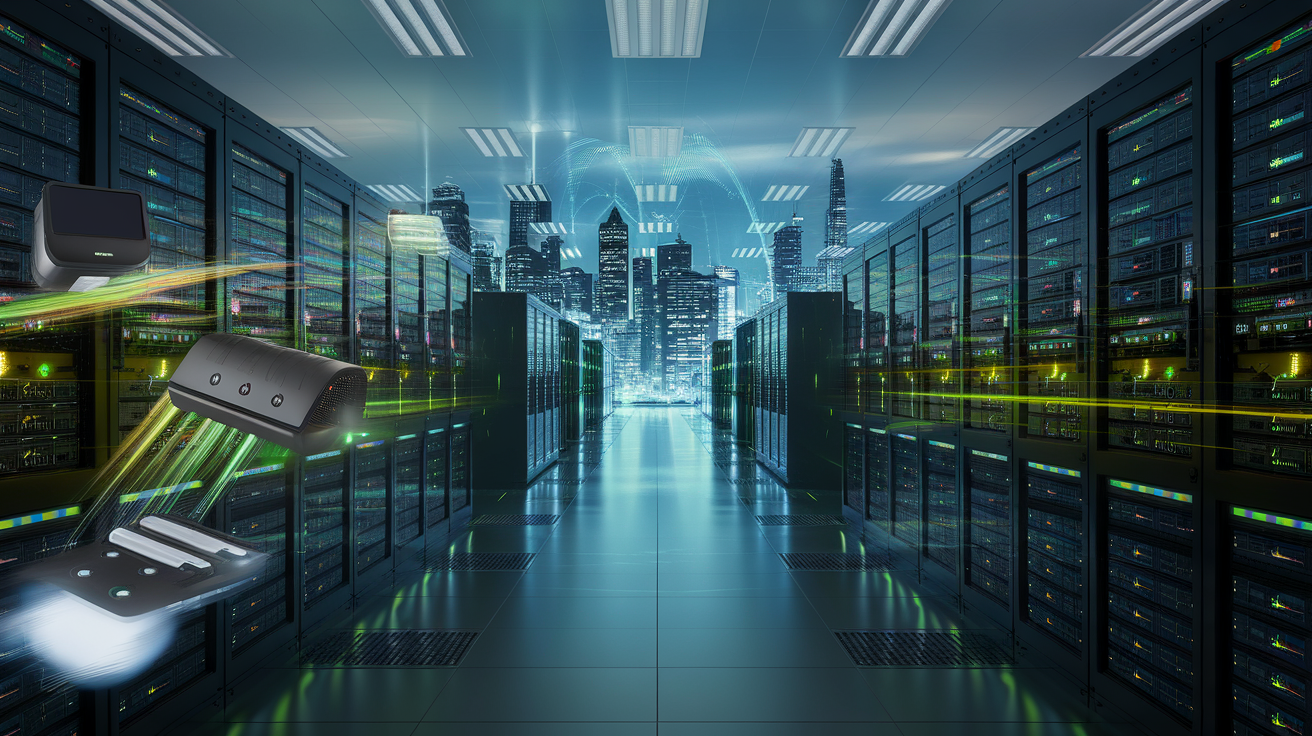
- Local Processing: Reduces latency by processing data closer to the source.
- Data Reduction: Minimizes bandwidth usage by filtering unnecessary data before sending it to the cloud.
- Real-Time Decisions: Facilitates immediate insights and actions based on local data.
- Energy Efficiency: Lowers energy costs through optimized power consumption of edge devices.
- Scalability: Supports scaling by distributing workloads across multiple edge devices.
- Improved Analytics: Enhances overall data analysis with localized, context-aware processing.
- Fault Tolerance: Ensures continuous operation even with intermittent cloud connectivity.
- Security Boost: Local data processing reduces the risk of data exposure during transmission.
FAQs on the Role of Edge AI in Enhancing Cloud Computing Efficiency
What is Edge AI? Edge AI refers to the deployment of artificial intelligence models directly on local edge devices, allowing for real-time data processing and analysis without the need to rely solely on central cloud servers.
How does Edge AI improve cloud computing efficiency? By processing data locally, Edge AI minimizes the amount of information that needs to be sent to the cloud, which in turn reduces latency and enhances network efficiency.
What are the benefits of using Edge AI? Edge AI provides numerous advantages such as reduced energy costs, improved data privacy, and faster response times, making it a superior option for various applications.
How does cloud computing support Edge AI? Cloud computing provides the necessary computational power, storage capacity, and scalability to manage the large volumes of data generated by Edge AI devices, thereby enhancing their overall performance.
What are some use cases for Edge AI? Edge AI can be utilized in various fields, including healthcare for real-time patient monitoring, in smart cities for traffic management, and in manufacturing for predictive maintenance.
Is Edge AI suitable for all organizations? While Edge AI offers significant benefits, the suitability largely depends on the specific needs of an organization, such as the need for real-time processing and the existing infrastructure.
How does Edge AI and cloud computing work together? They complement each other by allowing Edge AI to perform immediate tasks with local data processing while the cloud handles long-term data analysis and management.
What challenges does Edge AI face in relation to cloud computing? Challenges include maintaining security during data transfers, ensuring device interoperability, and managing the potential for increased complexity in deployment.
Can Edge AI reduce the costs associated with cloud computing? Yes, by handling data processing at the edge, organizations can significantly decrease the amount of data transferred to the cloud, leading to lower storage and bandwidth costs.
What future trends are anticipated in Edge AI and cloud computing? Ongoing advancements in machine learning and IoT technologies will likely enhance the integration of Edge AI and cloud computing, making systems more efficient and responsive.