Edge AI represents a significant advancement in the realm of technology, particularly in its ability to enhance cloud computing. By enabling real-time data processing on local devices, Edge AI alleviates many challenges associated with traditional cloud infrastructures, such as latency and bandwidth constraints. This integration not only optimizes data handling but also improves the way applications interact with vast datasets stored in the cloud. As a result, organizations can achieve faster decision-making, greater operational efficiency, and an enhanced user experience, positioning Edge AI as a transformative force in the ongoing evolution of cloud services.
Edge AI represents a significant step forward in how we process data. By allowing artificial intelligence to function directly on local devices rather than relying solely on the cloud, this technology not only reduces latency but also addresses some of the inherent limitations of traditional cloud computing. In this overview, we’ll explore how the integration of Edge AI and cloud services creates a more efficient ecosystem for real-time data processing.
Understanding Edge AI
At its core, Edge AI involves deploying artificial intelligence models directly onto devices situated at the ‘edge’ of the network. This means that AI applications can analyze data in real-time, right where it’s generated, without needing to send all that information back to the cloud. As a result, you see faster decision-making processes and enhanced responsiveness—key factors in today’s fast-paced digital landscape.
Imagine you are using a smart camera that recognizes faces. Instead of sending each captured image to a remote server for analysis, this camera can process the images right away. This approach reduces the bandwidth needed and minimizes the time required to obtain results.
Cloud Computing and Edge AI: A Symbiotic Relationship
While Edge AI brings significant advantages, it is worth highlighting that cloud computing plays a vital role in its effectiveness. Cloud services offer robust data storage, processing power, and the ability to update AI models as new information becomes available. This partnership enhances the capabilities of both technologies.
For instance, data gathered at the edge can be sent to the cloud for deeper analysis or larger-scale machine learning tasks. The cloud acts as a comprehensive manager, facilitating the storage of larger datasets and ensuring that edge devices remain updated with the latest model improvements.
Benefits of Integrating Edge AI with Cloud Solutions
The combination of Edge AI and cloud computing brings forth several benefits. One of the primary advantages is improved network efficiency. Since only the most essential data is transmitted to the cloud, there is a marked reduction in the volume of transferred data. This not only optimizes bandwidth usage but also enhances performance in environments where network connectivity may be limited or inconsistent.
Moreover, this synergy bolsters security measures. By processing sensitive information locally, the reliance on the cloud for every transaction diminishes, reducing potential vulnerabilities during data transfers. With Edge AI, even if the cloud is compromised, local processing can safeguard crucial operations.
Real-World Applications
Edge AI is already revolutionizing numerous sectors. In healthcare, devices equipped with Edge AI can monitor patients in real-time, providing immediate alerts for anomalies without overwhelming cloud systems. In manufacturing, smart sensors can optimize production lines by processing feedback on-site, allowing for quick adjustments. Many companies are leveraging these technologies for not just efficiency but also to strengthen operational security.
According to various sources, businesses are embracing Edge AI to enhance their cloud strategies without sacrificing performance. This transformation points toward a future where cloud computing isn’t just a distant hub but a pivotal part of a localized, responsive network.
The fusion of Edge AI with cloud computing is reshaping how we approach data processing. The capabilities offered by Edge AI, when supported by the cloud, lead to a significant evolution in efficiency and responsiveness, making way for smarter, more agile systems. As organizations continue to adopt these technologies, the journey toward seamless data integration and management promises to be both exciting and transformative.
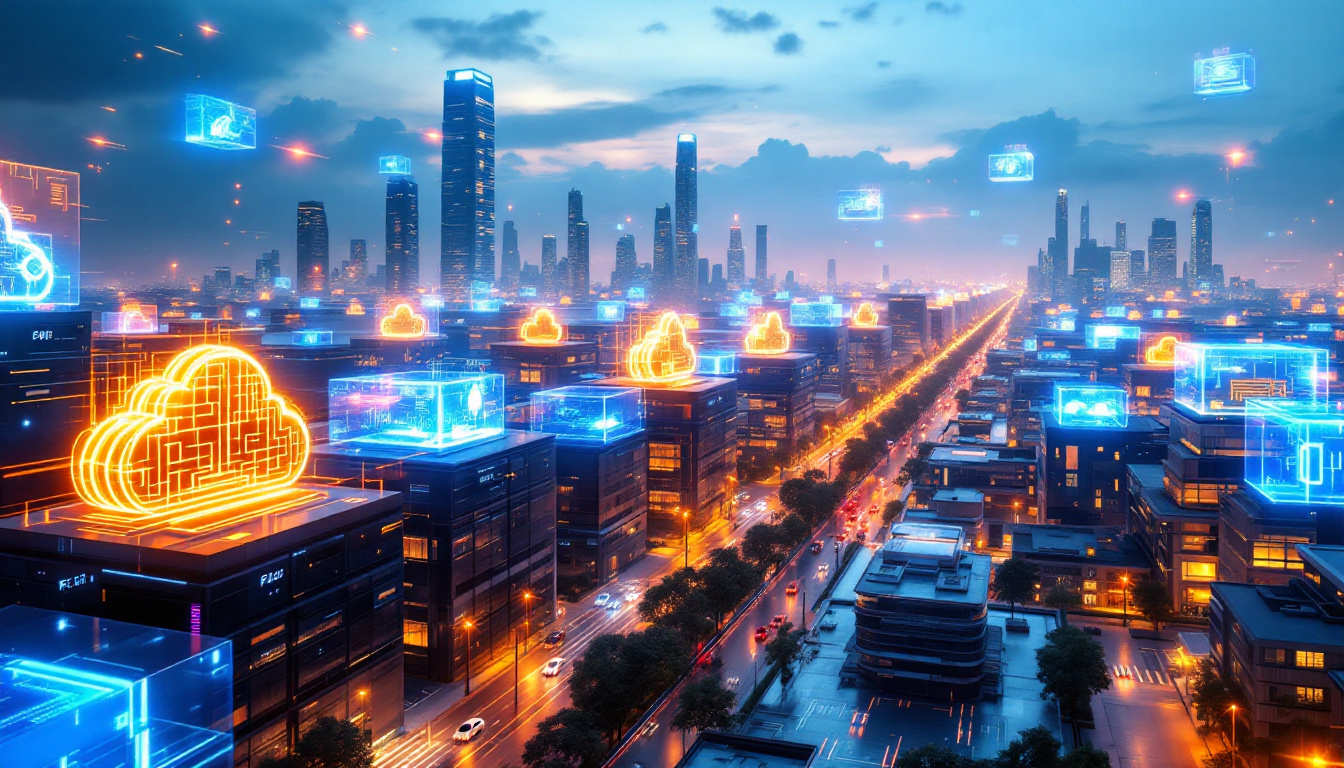
- Local Processing: Enables data to be processed on devices, reducing latency.
- Real-time Analytics: Facilitates immediate data insights for faster decision-making.
- Data Efficiency: Limits the volume of data sent to the cloud, conserving bandwidth.
- Model Updating: Supports continuous improvement of AI models via cloud resources.
- Enhanced Security: Processes sensitive data locally, reducing exposure in transit.
- Scalability: Adapts to increasing data loads by leveraging both edge and cloud capabilities.
- Reduced Costs: Minimizes cloud resource consumption by handling data at the edge.
- Hybrid Solutions: Combines on-premises and cloud resources for optimized operations.
- Decentralized Intelligence: Distributes processing, enhancing operational resilience.
- Interoperability: Simplifies integration of diverse devices with cloud systems.